Machine learning has emerged as a revolutionary technology across almost all sectors in the past few years. Within fintech, machine learning algorithms provide creative solutions that push the boundaries of what was previously possible. As more high-quality training data becomes accessible and processing power improves dramatically, fintech machine learning applications will continue to grow significantly.
This article examines the top eight ways financial technology businesses are currently using machine learning to disrupt old systems, improve client experiences, and increase efficiency. Analyzing real-world instances demonstrates how sophisticated analytical tools help with anything from loan decisions to robo-advising while accelerating crucial new capabilities.
1. Credit Scoring & Risk Assessment
Improving traditional credit scoring models was one of the first fintech machine learning applications to be widely embraced. Whereas traditional credit agencies focus on a small number of factors, machine learning systems can evaluate thousands of data points about an individual’s financial history and activities over time.
This improved data analysis enables more tailored and predicted creditworthiness evaluations. Millions of applications who would have been refused owing to low credit histories are now approved through alternative lending platforms. Simultaneously, increased underwriting using machine learning protects lenders against higher-risk consumers while increasing approvals.
2. Fraud Detection
Machine learning algorithms are better at spotting tiny spending trends or account irregularities that indicate fraudulent activity than manual procedures. By processing massive transactional datasets, ML systems learn what “normal” spending habits look like across all clients and can detect even complex deception efforts in real time.
This enables continuous monitoring capabilities to detect fraudulent activity across financial institutions, payment processors, and fintech companies. Early discovery reduces liabilities while increasing user security and happiness through prompt resolution. Advanced neural networks can even self-update when new fraud strategies arise in order to remain ahead of thieves.
3. Investment Management
Robo-advisors that manage portfolios through algorithmic asset allocation rely heavily on supervised learning from previous financial market actions. Training on decades of historical returns enables robo-platforms to create highly efficient low-cost investing strategies suited to individual risk tolerances.
Continuously rebalancing assets in response to changing economic conditions and machine-predicted global events ensures that customers’ nest eggs develop sustainably for retirement or other savings objectives without the need for manual intervention. Machine investing monitoring also advantages retirement savings by charging lower costs than human advisors.
4. Trading & Capital Markets
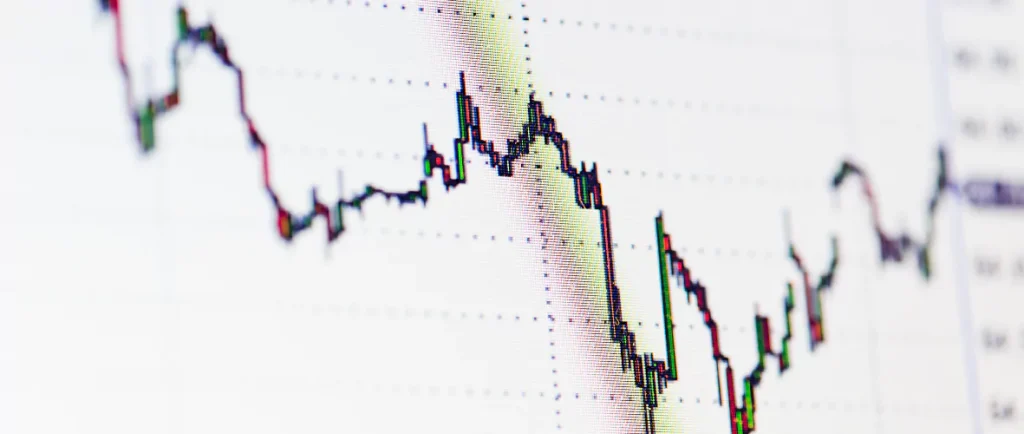
Algorithmic or quantitative trading dominates over 60% of equity markets daily volumes according to recent estimates. Behind the scenes, reinforcement learning powers autonomous trading bots to execute split-second strategies without direct human involvement. Through trial-and-error simulations, these agents optimize complex ordering strategies based on neural network-analyzed patterns arising from enormous order book datasets.
Top quantitative hedge funds deploy neural net patterns recognizing earnings surprises or detecting impending mergers/acquisitions ahead of Wall Street to gain lucrative trading edges. Fintech machine learning applications also helps investment banks remotely monitor distributed trading workflows across global markets for risk management.
5. Insurance Pricing & Underwriting
Similar to credit risk models, ML transforms insurance pricing by incorporating thousands more data points on individuals and their homes, cars, or health profiles to customize premiums appropriately. Where policies traditionally relied on just a handful of factors like age and location, machine-learned algorithms now account for lifestyle habits proven to impact one’s risk too.
This tailors insurance affordable for lower-risk applicants while protecting providers against high-risk customers. Simultaneously, enhanced ML-assisted underwriting powers entirely new usage-based, pay-as-you-drive auto policies and wellness-influenced medical plans promoting healthier living with rewards.
6. Personal Financial Management
Beyond maintaining essential banking needs, machine learning enables financial applications to help users optimize their everyday spending and saving habits. Pattern recognition in connected bank transactions teaches computers that regular charges, such as subscriptions, may be cancelled to free up cash. Neural networks can also find chances to earn more interest by moving money between accounts more efficiently.
Augmenting top productivity and budgeting tools, ML advisers guide users to financial independence through interactive gamified challenges, savings plan improvements, and cost advice tailored to their specific goals.
7. Customer Service & Support
Chatbots powered by natural language processing harness machine learning to assist customers with common inquiries 24/7 through conversational interfaces. Deep learning powers these virtual agents’ ability to resolve issues through thousands of training dialogues and scenario role plays enabling them to match user questions to predefined responses with human-level language comprehension.
Advanced neural networks proactively detect customer dissatisfaction by monitoring social media and survey language sentiment analysis. This guides live phone agents in precisely resolving pain points for improved retention. Chatbots augmented with human oversight also schedule appointments, complete basic account tasks and recommend products to improve lives through personalized financial assistance at scale.
8. Blockchain Analytics
While blockchain is best recognized for enabling cryptocurrency, its distributed ledger data representations provide a wealth of insight for machine learning algorithms. Partnerships between exchanges, miners, and fintech businesses use patterns discovered through training on petabyte transaction graphs to profile rogue actors, identify laundering rings, and anticipate currency volatility based on common investor herd behavior.
Neural networks detect imminent software vulnerabilities using codebase scanning before hackers exploit them. Overall, by evaluating such immutable, tamper-proof data, fintech machine learning applications promise to bring unparalleled supervision, protecting finances in an era of digital value transfers and assisting blockchain in fulfilling its promise as a force for transparency and good.
Fintech Machine Learning Applications
Fintech machine learning applications, as illustrated by these eight significant areas, shapes the future of financial services through customization, efficiency, security, and innovation. Early adopters enjoy a competitive edge, while customers benefit from services that are personalized, optimized, and protected thanks to data-driven intelligence infusion. The possibilities appear infinite as computers continue to improve people’s lives by learning directly from their own experiences on unprecedented proportions. Finance has never been more tailored or intuitively aided, demonstrating machine learning’s potential to alter every business it touches.